What we’ve learned so far about AI-powered functionalities for learning and assessment:
- Improves outcomes, increases engagement and knowledge retention, decreases training time, and more (learn more here)
- Increases ROI on learning initiatives, produces better business outcomes, and boosts employee engagement and retention (learn more here)
- These positive outcomes are a result of several components working together to form enhanced learning and assessment experiences: algorithms, machine learning, Gen AI, Large Language Models, Natural Language Processing, Data Learning Analytics (Learn more here)
So, AI is good for learning assessment. It’s good for organizational performance. This is made possible because of its ability to analyze a lot of data, really fast, and use its analysis to produce unique, tailored learning paths, based on the needs of each employee. Great. AI seems like it presents a clear value add in the skills assessment arena.
But like anything that sounds this good, it’s not without its challenges. Before jumping in, it’s worth pausing to look at the risks that come with using AI in learning assessment—and what we can do to manage them. What should L&D leaders be watching out for, and how can they put the right guardrails in place?
AI Assessment Risks at a Glance
The information below breaks down our research findings on key risks associated with AI-powered assessment—along with real-world examples of what those risks can look like in practice for L&D teams.
AI Component
Associated Risk (brief)
Real-World Scenario
Algorithms
Bias & Discrimination – Models trained on skewed data can systematically disadvantage certain groups.
An AI tool used to evaluate leadership potential consistently scores women lower than men due to biased historical data—resulting in fewer women being shortlisted for leadership development programs.
Machine
Learning
Inaccurate or Unreliable Assessments – Systems can “hallucinate” or misinterpret inputs without human checks.
In a manufacturing firm, a machine learning model misclassifies several proficient workers as “not qualified” during a safety certification assessment. As a result, those workers are pulled from emergency response teams and miss crucial hands-on training—leaving actual gaps in preparedness when an incident occurs.
Learning Data Analytics
Data Privacy & Security – Collecting sensitive user data without airtight controls risks breaches and regulatory fines.
A financial organization’s analytics platform leaks assessment scores in a misconfigured cloud repository—triggering a GDPR investigation.
GenAI
Overreliance & Reduced Oversight – Blind faith in AI decisions can miss context only humans catch.
An L&D team uses a GenAI tool to automatically generate assessment questions and grade responses for a technical upskilling program. Without sufficient human review, the AI produces several poorly framed questions with ambiguous wording and inaccurate answer keys. Learners become frustrated when correct responses are marked wrong, undermining their confidence and trust in the assessment process. The issue goes unnoticed for weeks, impacting completion rates and learner satisfaction.
NLPs + LLMs
Compliance & Regulatory Risk – Failing to keep pace with evolving fairness/transparency laws can incur big penalties.
A financial services firm uses an NLP tool to score written responses in its FINRA-mandated compliance training. The tool flags certain responses as non-compliant but lacks a transparent audit trail for how those decisions were made. During a FINRA audit, the firm is unable to demonstrate consistent, explainable evaluation criteria—raising red flags about fairness and regulatory adherence.
AI Component
Algorithms
Associated Risk (brief)
Bias & Discrimination – Models trained on skewed data can systematically disadvantage certain groups.
Real-World Scenario
An AI tool used to evaluate leadership potential consistently scores women lower than men due to biased historical data—resulting in fewer women being shortlisted for leadership development programs.
Machine Learning
Associated Risk (brief)
Inaccurate or Unreliable Assessments – Systems can “hallucinate” or misinterpret inputs without human checks.
Real-World Scenario
In a manufacturing firm, a machine learning model misclassifies several proficient workers as “not qualified” during a safety certification assessment. As a result, those workers are pulled from emergency response teams and miss crucial hands-on training—leaving actual gaps in preparedness when an incident occurs.
Learning Data Analytics
Associated Risk (brief)
Data Privacy & Security – Collecting sensitive user data without airtight controls risks breaches and regulatory fines.
Real-World Scenario
A financial organization’s analytics platform leaks assessment scores in a misconfigured cloud repository—triggering a GDPR investigation.
GenAI
Associated Risk (brief)
Overreliance & Reduced Oversight – Blind faith in AI decisions can miss context only humans catch.
Real-World Scenario
An L&D team uses a GenAI tool to automatically generate assessment questions and grade responses for a technical upskilling program. Without sufficient human review, the AI produces several poorly framed questions with ambiguous wording and inaccurate answer keys. Learners become frustrated when correct responses are marked wrong, undermining their confidence and trust in the assessment process. The issue goes unnoticed for weeks, impacting completion rates and learner satisfaction.
NLPs + LLMs
Associated Risk (brief)
Compliance & Regulatory Risk – Failing to keep pace with evolving fairness/transparency laws can incur big penalties.
Real-World Scenario
A financial services firm uses an NLP tool to score written responses in its FINRA-mandated compliance training. The tool flags certain responses as non-compliant but lacks a transparent audit trail for how those decisions were made. During a FINRA audit, the firm is unable to demonstrate consistent, explainable evaluation criteria—raising red flags about fairness and regulatory adherence.
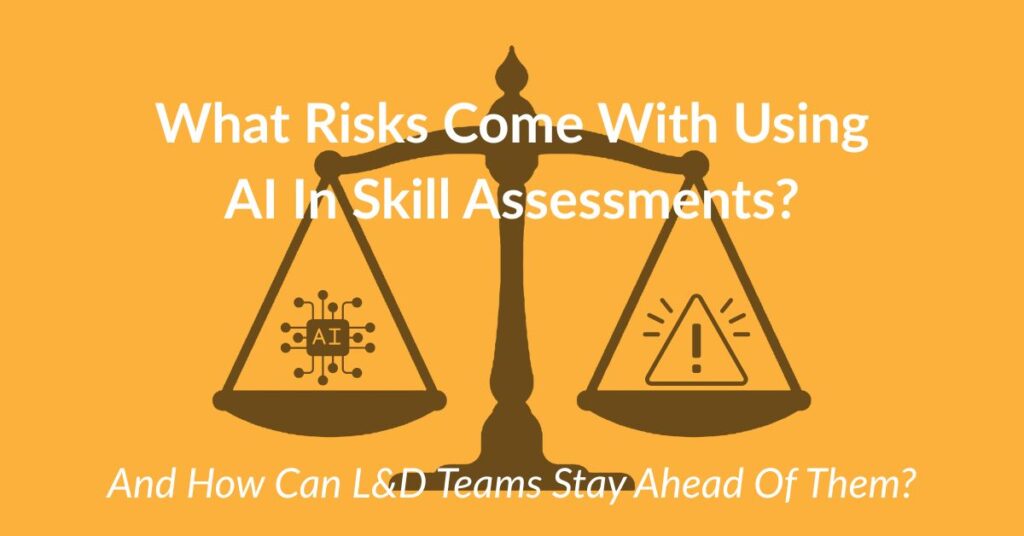
Mitigating Risks
AI brings a lot to the skills assessment table, and incorporating it into your learning strategy is more likely a question of “when and how”, than “if”. That being said, our research shows that it´s important to consider all of the possible implications of its implementation in order to safeguard the organization, its data, and their employees. We’ve compiled a list based on our research of few practical ways to do just that:
1. Mitigate Algorithmic Bias
- Use diverse, representative training data.
- Regularly audit models (These tools can help: IBM’s AI Fairness 360, LIME, or SHAP)
- Follow industry ethical guidelines (e.g., IEEE, ACM).
2. Ensure Data Privacy & Security
- Anonymize and encrypt employee data.
- Use role-based access controls to limit exposure.
- Confirm vendor compliance with privacy laws (e.g., GDPR, CCPA, HIPAA, EU AI Act).
Clearly explain how AI is used and what data is collected and provide channels for feedback.
3. Maintain Human Oversight
- Use human reviewers for critical decisions like promotions, compliance training content, or certifications.
- Leverage explainable AI tools so employees understand how assessments are scored. (These tools can help you: ELI5, AIX360, Interpret ML, Anchors, Google Vertex, InterpretML – Microsoft)
- Test before you scale and ensure that edge cases are handled reliably.
- Track KPIs like accuracy, fairness, and learner satisfaction.
4. Upskill the L&D Team
- Offer AI literacy and ethics training.
- Teach teams how to use and evaluate generative AI responsibly